Welcome to the second edition of the second season of ZtoA Pulse.
In this digital age, where every keystroke and transaction generates data, harnessing its power is pivotal. At the heart of this transformation lies the amalgamation of insights and expertise, where the pioneers of knowledge intersect with the trailblazers of technology. That’s precisely what this edition encapsulates— a newsletter that amalgamates the expertise of Zuci Systems’ thought leaders with insights from one of the Gartner’s recent data and analytics reports.
With a keen eye on the horizon, we’ve crafted this newsletter to decode the future of data & analytics using the lenses provided by Gartner. Their recent report, “Over 100 Data and Analytics Predictions Through 2028” (link provided below), acts as a beacon for organizations to fool-proof their data and analytics framework.
This newsletter will dive deep into four predictions, seeking our expert’s insights and unraveling its layers to enrich the discourse. Together, we will challenge assumptions, spark conversations, and build a bridge between the theoretical and practical facets of Gartner’s data and analytics predictions.
Introducing Zuci System’s Experts:
1) Kalyan Allam
Hello all, I am Naresh Kumar, Lead Marketing Strategist at Zuci Systems. Let’s delve into the four Gartner’s predictions that help shape organizations’ data and analytics game!
Prediction 1 – By 2026, 50% of BI tools will activate their users’ metadata, offering insights and data stories with recommended contextualized journeys and actions.
Moving to Kalyan Allam
Naresh: What are the main benefits that organizations and users can expect from BI tools that offer recommended contextualized journeys and actions?
Kalyan: Typically, decision makers consistently seek answers to recurring questions, while the data itself undergoes constant change. The narrative they aim to construct from the data shifts as circumstances evolve. So, the desire to obtain customized insights has been hard, to achieve the goal for organizations.
However, extracting these insights from various data sources remains an obstacle. Such difficulties endure because each person engages with a particular set of data comprehends it in their own way, resulting in different narratives. This scenario emphasizes the importance of individualized insights, which are incredibly valuable. To achieve this goal, organizations need to gather users’ metadata.
Developing systems that document users’ analytical journeys and comprehend the intent behind each inquiry is essential. This concept of actor-based analytics will significantly benefit organizations in numerous ways.
Naresh: Could you shed some light on how organizations benefit from actor-based analytics and customized insights?
Kalyan: Sure, Naresh. That’s an excellent question.
Some of the ways organizations can benefit from actor-based analytics and customized insights are:
1. Elevated User Engagement: The provision of tailored insights fosters a heightened level of engagement among users, enticing them to actively participate and interact more frequently with the business intelligence (BI) tool. This enhanced engagement stems from the relevance and specificity of the insights, resonating more effectively with individual user needs and objectives.
2. Mitigated Cognitive Overload: By furnishing personalized insights, organizations reduce cognitive strain by delivering information precisely aligned with users’ interests. This approach enables users to focus their attention on decision-making processes.
3. Optimized Resource Allocation: Gaining insights into users’ analytics journeys empowers organizations to allocate resources for optimal returns. By discerning the patterns and preferences that emerge from user interactions, organizations can channel investments towards areas that promise the most significant impact and value, thus driving efficiency and maximizing resource utilization.
Naresh: How do these BI tools identify and recommend relevant contextualized journeys and actions for users? What algorithms or methodologies are commonly used to tailor insights to individual needs?
Kalyan: Currently, there is a gap in the availability of ready-made solutions that can deliver customized insights to users. To identify a contextualized journey within business intelligence tools (BI) and provide tailored insights to users, it is time-pressing to concentrate on two pivotal aspects.
Naresh: Could you take us through the two aspects?
Kalyan: Firstly, it is vital to capture the users’ metadata. This entails gathering information encompassing the user’s roles and responsibilities, the specific data sets they access, and the questions they pose. By holistically capturing these elements, organizations can form a comprehensive picture of individual user profiles, enabling the creation of insights that resonate directly with their unique needs and requirements.
Secondly, the convergence of machine learning (ML) technologies (particularly Large Language Models such as OpenAI’s GPT and LLMs offered by platforms like Hugging Face) with BI tools like Tableau or Power BI can ease the process. These LLMs possess the remarkable capability to contextualize users’ journeys by capturing their specific demands and intents. To operationalize this model, organizations should establish a system wherein the analytical journey commences with prompts. This prompts-based approach facilitates the capture of users’ metadata, enabling the system to continually learn and refine its insights delivery based on the evolving user interaction patterns.
By utilizing these strategies, organizations can pave the way for a transformative approach to personalized insights, integrating advanced ML technologies and BI tools, and ultimately enhancing the user experience by providing precisely tailored analytical solutions.
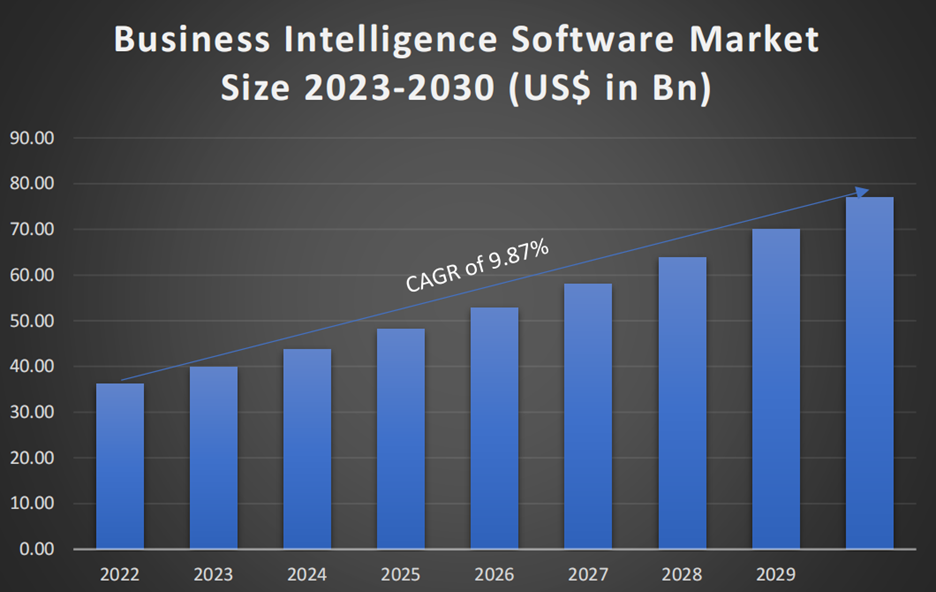
According to SNS Insider Research, the Business Intelligence Software Market size was valued at US$ 36.27 Bn in 2022 and is projected to reach US$ 77.03 Bn by 2030, with a growing healthy CAGR of 9.87% over the Forecast Period 2023-2030.
Prediction 2: Through 2026, more than 60% of threat detection, investigation and response (TDIR) capabilities will leverage exposure management data to validate and prioritize detected threats, up from less than 5% today.
Moving to Janarthanan Poornavel
Naresh: How do AI and ML play a role in analyzing exposure management data for threat detection and response? What are the key machine learning algorithms and techniques used in this context?
Jana: I would like to bring up one of the success stories highlighting the seamless integration of AI and ML, with respect to exposure data management and TDIR, in one of our recent projects to combat the complex challenge of bank check fraud.
Considering the np-hard problem, finding a perfect solution in a reasonable time was incredibly challenging. In our case, the complexity was compounded by the exponential increase in the volume of transactions. As you can imagine, the sheer number of transactions to analyze could quickly overwhelm traditional approaches, making it difficult to detect potentially fraudulent activities involving transaction history, account behavior, and patterns.
However, integrating AI and ML helped us analyze vast amounts of data, recognize patterns, and adapt to evolving threats, empowering us to create a formidable defense mechanism against check fraud. With the successful implementation of exposure management-driven threat detection, AI algorithms swiftly assessed transactional behavior to identify anomalies that might indicate fraudulent activities targeting “x number” of pesos.
Naresh: Jana, you have provided insights into how AI and ML were applied to address the check fraud scenario. Could you explain how Machine Learning offers possible solutions for exposure data management in the context of threat detection?
Jana: Sure, Naresh. Machine Learning brings robust solutions to the table for threat detection. Let me break down a couple of crucial aspects, i.e., pattern recognition and anomaly detection.
- Pattern Recognition: Machine Learning algorithms excel at pattern recognition. Through rigorous training on a diverse dataset of handwritten checks, the ML models become adept at distinguishing between legitimate and fraudulent alterations. This enhanced pattern recognition enhances our sensitivity to even the most subtle anomalies.
- Anomaly Detection: ML thrives on detecting anomalies—irregularities that might go unnoticed by traditional methods. Exposure management data encompasses a treasure trove of contextual information. We leverage ML algorithms to uncover hidden correlations and deviations that indicate potential threats.
Naresh: Jana, your success story highlights the power of AI and ML in threat detection. Could you walk me through some specific ways AI and ML paved the way for this success?
Jana: That’s an excellent question.
Advanced Deep Learning Algorithms and Inference Service Stack played a significant role in achieving fraud detection. Let me take you through this step by step:
Step 1 – Our team employed state-of-the-art deep learning algorithms to tackle this complex challenge. These advanced algorithms, driven by machine learning, recognize patterns and anomalies within vast datasets. By training these models on a diverse array of handwritten checks, we were able to enhance sensitivity and specificity, enabling the identification of even the most subtle fraudulent alterations.
Step 2 – Since the bank has over 1000 branches, we established an innovative inference service stack to minimize this check fraud across all the branches. This architecture allowed us to process an immense volume of checks efficiently, maximizing the capabilities of our deep-learning models. The combination of AI and efficient infrastructure proved to be a winning formula.
Naresh: That’s a smart approach, Jana. It’s impressive how you leveraged cutting-edge algorithms and a scalable infrastructure to combat the challenge effectively.
Jana: In the context of exposure management data analysis for threat detection and response in check fraud, AI and ML have proven to be transformative catalysts. Our deep learning, infused with the power of machine learning algorithms (Yolo and Faster CNN), enables us to discern fraudulent patterns with unprecedented accuracy. Moreover, our sophisticated inference service stack ensures that this advanced technology can be deployed efficiently across the network.
Well, Naresh, so, to conclude, I align with Gartner’s insights into the evolving threat detection landscape. Their prediction that over 60% of threat detection capabilities will leverage exposure management data by 2026 resonates with our approach. By incorporating exposure management data, I anticipate an impressive growth of 15% enhancement in the TDIR, which is 5% today.
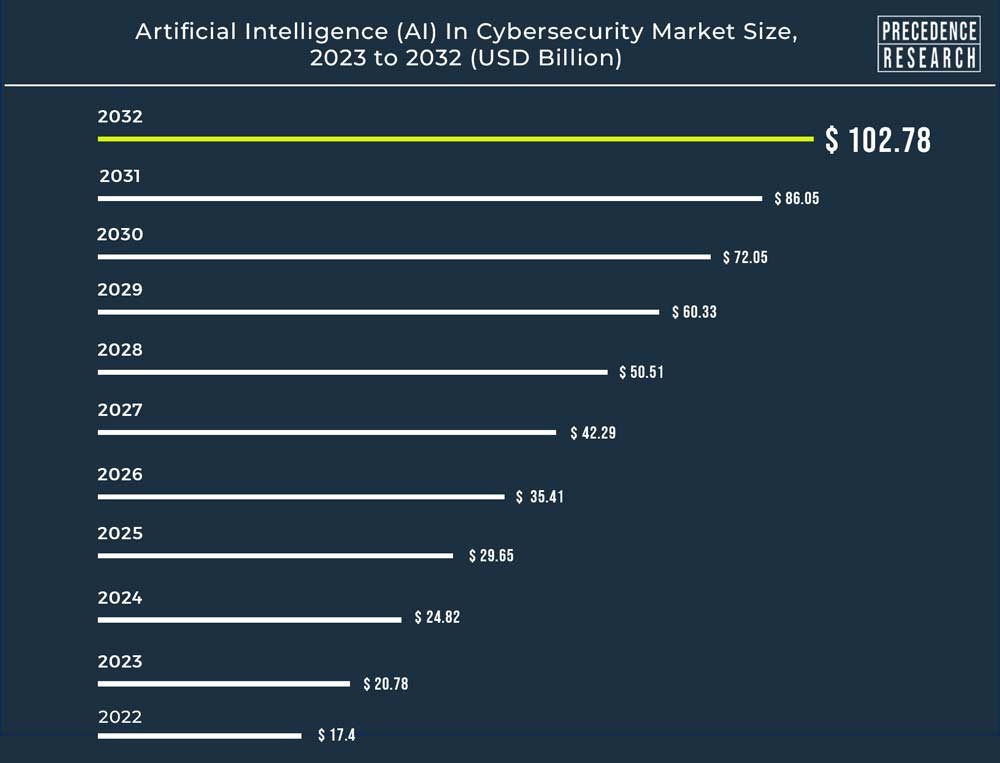
The global artificial intelligence (AI) in cybersecurity market size was evaluated at USD 17.4 billion in 2022 and is expected to hit around USD 102.78 billion by 2032, growing at a CAGR of 19.43% between 2023 and 2032.
Prediction 3: By 2027, 50% of enterprise software engineers will use ML-powered coding tools, up from less than 5% today.
Moving to Sridevi Ramasamy
Sridevi: It is evident that the integration of AI and ML models directly into the application development process will fundamentally reshape how businesses operate and innovate.
One of the most compelling aspects of embedding AI and ML models into application development is their potential to revolutionize data analysis and trend prediction. By leveraging these technologies, businesses can extract valuable insights from their existing data, make informed decisions, and identify emerging trends in their respective industries.
While this technological shift represents a significant advancement, it is essential to acknowledge that this transformation cannot happen overnight. The journey towards effectively implementing AI and ML in software engineering will require continuous learning, collaboration, and adaptation from engineering teams. ML tools assist developers in navigating the complexities of AI integration and ensuring that the end products are robust, efficient, and equipped with cutting-edge capabilities.
Naresh: Could you share some benefits?
Sridevi: Sure, Naresh. Let me outline a couple of ways through which organizations can derive benefits:
- Decision-Making Ability
ML-powered tools can incorporate Natural Language Processing (NLP) to understand and process natural language search queries. This feature enables developers to enter search queries in a manner that closely resembles human language, thereby elevating the overall user experience.
Advanced Search can benefit from ML-generated contextual suggestions. These suggestions might include relevant code examples, libraries, or functions that are closely related to the search query, helping developers explore different solutions.
- Overall Business Growth
The predictive capabilities of ML-powered applications significantly bolster business growth. Organizations can anticipate trends, market shifts, and customer behaviors. Armed with this foresight, they can proactively adjust strategies to capitalize on emerging opportunities and mitigate potential risks. A couple of use cases are mentioned below:
- Collect user feedback and usage analytics to understand how well the integrated AI/ML features work.
- Enable the application to perform real-time inference by feeding new data to the integrated models.
Through the utilization of data-driven decision-making and predictive capabilities, these applications propel business strategies to unprecedented heights. Besides, the role of Product Managers evolves as ML-powered apps suggest product features to facilitate fast-paced growth.
Naresh: How do machine learning-powered tools enhance the productivity of software engineers?
Sridevi: By leveraging these tools, engineering teams can tap into the power of ML, allowing engineers to incorporate ML capabilities into their projects effectively.
- ML-powered coding tools can analyze code and provide suggestions for optimization, such as improving algorithm efficiency, reducing memory usage, and minimizing resource consumption.
- ML-powered coding tools can automate repetitive and time-consuming tasks like code generation, completion, and debugging.
Integrating AI/ML models into application development requires a multidisciplinary approach involving developers, data scientists, domain experts, and UI/UX designers. Successful integration can lead to applications that provide enhanced functionality, better user experiences, and data-driven insights for decision-making.
Prediction 4: By 2026, organizations adopting active metadata practices will increase to 30% to accelerate automation, insight discovery, and recommendations.
Moving to Rajkumar Purushothaman
Naresh: For organizations considering adopting active metadata practices in data and analytics, what are the potential challenges or hurdles they might encounter during implementation?
Raj: Well, some challenges could be:
- Data silos
- Lack of data consistency/quality
- Lack of metadata accuracy
- Lack of feasibility to integrate with analytics tools
Though each organization encounters different challenges, these are some critical challenges most organizations experience.
Naresh: Could you share some best practices that can help overcome these challenges?
Raj: Sure, Naresh.
I would like to break this down into best practices and ideal approaches to overcome the challenges.
Challenge 1 – Data Silos
Best Practices to Eliminate Data Silos
- Implement a unified data governance framework
- Utilize data integration tools to connect disparate data sources
- Apply standardized metadata tags to ensure consistency
Ideal Approach to Overcome the Challenge
- Establish a centralized data governance team to create and enforce metadata standards.
- Utilize industry-leading tools such as Informatica Metadata Manager to create a unified metadata repository.
- Standardize naming conventions and metadata attributes across data sources.
Challenge 2 – Lack of Data Consistency/Quality
Best Practices to Ensure Data Quality
- Implement data quality checks and validation rules
- Utilize active metadata to track changes in data quality metrics
- Establish data stewardship roles and responsibilities
Ideal Approach to Overcome the Challenge
- Implement automated data quality rules using standard tools.
- Deploy active metadata triggers to capture changes in data quality metrics such as duplicate records, missing values, or outliers.
- Appoint data stewards responsible for monitoring and improving data quality.
Challenge 3 – Lack of Metadata Accuracy
Best Practices
- Implement automated metadata validation processes
- Utilize data lineage to track changes and lineage accuracy
- Conduct regular metadata audits and quality checks
Ideal Approach to Overcome the Challenge
- Create automated processes that validate metadata attributes against predefined rules, ensuring consistency and accuracy.
- Utilize data lineage tracking to verify the accuracy of metadata by comparing it with the actual data flow.
- Regularly conduct metadata quality audits to identify and rectify inconsistencies or inaccuracies.
Challenge 4 – Lack of Feasibility to Integrate with Analytics Tools
Best Practice
- Choose metadata solutions that integrate with popular analytics platforms
- Develop metadata connectors for seamless data exchange
- Provide documentation and support for analytics tool integration
Ideal Approach to Overcome the Challenge
- Select metadata solutions that integrate with widely used analytics platforms such as Tableau and Power BI.
- Develop custom metadata connectors to enable seamless data exchange between the metadata repository and analytics tools.
- Provide clear documentation and user guides to help analysts integrate and utilize metadata within their existing workflows.
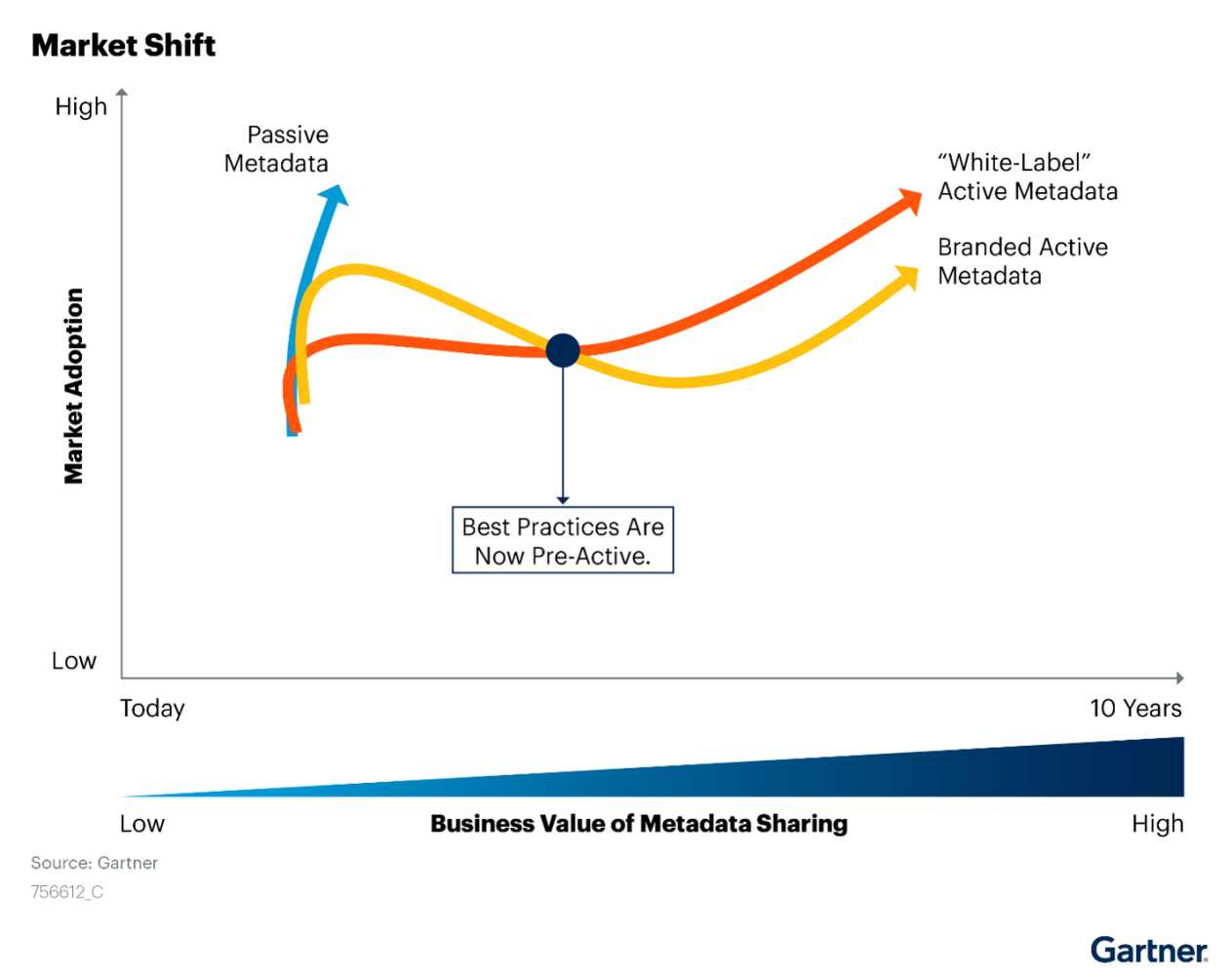
Naresh: Could you explain some potential automation use cases utilizing active metadata tools?
Raj: That’s a good question.
In the data and analytics automation process, active metadata tools play a crucial role, and here I am highlighting some use cases.
- Automated Data Ingestion and Integration
- Real-Time Analytics and Dashboards
- Automated Data Quality Checks
- Predictive Model Refreshes
- Automated Data Lineage and Impact Analysis
Naresh: Could you take me through these use cases?
Raj: Sure, I would like to share some classic approaches and solutions to each of these use cases.
Use Case 1 – Automated Data Ingestion and Integration
Approach:
Active metadata tools continuously monitor data sources for changes and trigger automated ingestion and integration workflows. They capture metadata about data formats, structures, and relationships to ensure accurate and seamless data integration.
Solution:
Use industry-standard tools to create data pipelines that automate the extraction, transformation, and loading (ETL) processes. Active metadata tracks changes and metadata attributes, ensuring real-time data integration across diverse sources.
Use Case 2 – Real-Time Analytics and Dashboards
Approach:
Active metadata enables real-time updates for analytics dashboards and reports by integrating with visualization tools. It ensures that dashboards display the most current insights as data changes.
Solution:
Leverage tools like Tableau or Power BI to build dynamic dashboards. Active metadata integrates with these tools, triggering automatic data refreshes whenever the underlying data changes. This approach ensures that decision-makers access up-to-the-minute insights.
Use Case 3 – Automated Data Quality Checks
Approach:
Active metadata collaborates with data quality tools to trigger automated data quality checks based on predefined rules. It captures metadata about data quality metrics and anomalies.
Solution:
Utilize industry-standard data quality tools like Informatica Data Quality. Active metadata interacts with the tools to initiate automated data validation processes, ensuring data accuracy and consistency.
Use Case 4 – Predictive Model Refreshes
Approach:
Active metadata monitors changes in data inputs and triggers automated retraining of predictive models. It captures metadata about model versions and data dependencies.
Solution:
Utilize machine learning platforms like TensorFlow, scikit-learn, or cloud native offerings. Active metadata detects data changes, triggers model retraining, and updates metadata to reflect the latest model versions, ensuring accurate predictions.
By utilizing these approaches and leading active metadata tools, organizations can achieve efficient and effective automation in various data and analytics processes. These tools enhance agility, accuracy, and decision-making by harnessing the power of active metadata.
Originally featured in our LinkedIn Newsletter, ZtoA Pulse.
Want to connect with our experts? Leave your details and we will get back to you.